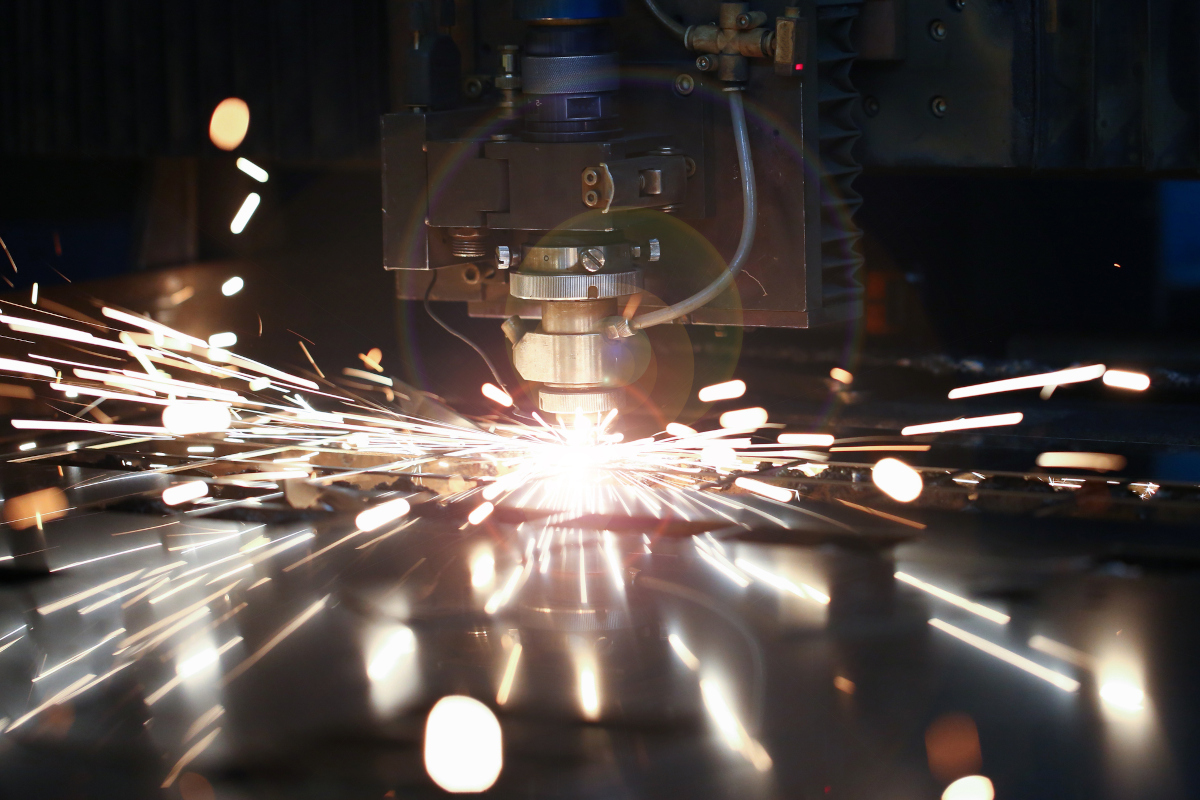
By Nils Klute, Specialist IT Editor and IoT Project Manager at eco – Association of the Internet Industry V.
Optimizing production processes, reducing waste, and keeping plants available – what artificial intelligence (AI) can achieve in manufacturing is not yet widely known in industry. Dr. Gunar Ernis from the Fraunhofer Institute explained how SMEs and AI can successfully come together in a Service-Meister webinar.
Anyone who has children knows: There’s no chance of uninterrupted sleep without sufficiently absorbent and therefore dry diapers. What makes life more pleasant and easier for parents and children is a major challenge for the industry. The production of so-called superabsorbent polymers is technologically demanding. In order to generate results with the necessary absorbency, the processes must be optimally controlled. The finest deviations in raw material quality, slight temperature fluctuations in the factory, and machine characteristics that vary according to throughput make things difficult. The solution is provided by Big Data and AI. Machine Learning (ML) simulates manufacturing processes and controls procedures in real time. It can balance out fluctuations and consistently produce optimal hygiene products, as the VDI status report Opportunities with Big Data records. “What AI and machine learning can achieve in manufacturing is not yet widely known in the industry,” said Dr. Gunar Ernis. The head of the Industrial Analytics business unit at the Fraunhofer Institute for Intelligent Analysis and Information Systems (IAIS) explained in a webinar by Service-Meister how data can drive the optimization of industrial production.
SMEs working on data readiness
A survey of experts conducted by the Technology Foundation Berlin in June 2020 confirmed that only a minority of SMEs used AI systems. What could be the reason for that? “Although the companies are very interested in practical applications, they lack the necessary data science know-how”” said Ernis. To get started, companies need to work on their data readiness. Heterogeneous data landscapes, information silos, and monolithic IT systems that cannot scale slow down the use of AI. The Fraunhofer lead project Machine Learning for Production solves challenges like these: “ML4P provides SME users with tools suitable for industry for the efficient use of ML methods and supports companies with a process and role model,” said Ernis.
Which processes do I want to support with AI? Which data sources and technologies are required to do so? And how do investments pay off? Ernis: “We define goals, clarify questions, prepare the implementation and, in addition, involve all project participants.” Regardless of whether they are process, IT security, or automation engineers – when AI comes into play, their know-how must ultimately flow to an ML specialist who will later program the models.
Maturity level of own data management is decisive
“The next step is to provide the data basis for the analyses,” said Ernis. “Depending on the maturity level of your own data management, more or less work is required.” While some companies have so far used data only sporadically to make ad hoc decisions at project level, others are already relying on a broad information base to continuously improve and analyze processes. “Whether log files, process and status parameters – the more information companies collect, the better,” says Ernis. Ideally, companies should store all data in a data lake. Heterogeneous information can be stored and processed here and metadata can also be extracted.
When data collection, AI, and ML finally come together in the concrete project, production can not only be simulated, planned, and optimized on the basis of data, but also controlled in real time. ML models predict how, for example, varying target properties of raw materials or deviating process parameters affect the target quality of a product. The results of the AI analyses can then be used to take countermeasures. “If the machine is able to control itself intelligently and compensate for fluctuations, it can optimize manufacturing.” The result: Maximum quality, minimum waste.
AI in SMEs: Numerous use cases, enormous potential
“There are numerous use cases that confirm the enormous potential of AI,” said Ernis. And a study by eco – Association of the Internet Industry underlines this: If AI is also used extensively in SMEs, a growth in gross domestic product of over 13 percent by 2025 (compared to 2019) is realistic, which corresponds to a total potential of around 488 billion Euros.
You liked this article? Then subscribe to our newsletter and receive regular updates on similar topics and the Project Service-Meister and discuss with us about this and similar exciting topics in our LinkedIn Group.