In numerous sectors of the German economy, a fundamental change in the creation of value from products to services can currently be observed:
In the past, companies have been able to generate high sales and margins in particular by selling high-quality industrial equipment (e.g. printing presses).
In recent years, however, especially due to an increasing number of market participants from countries with lower production costs, price pressure has increased significantly and in many sectors the margins of this traditional business model have been significantly reduced and, in some cases, even eliminated completely.
In addition, customers are increasingly shying away from investing in expensive industrial plants, as future repairs and maintenance are uncertain, and are increasingly preferring “operator models” which contractually guarantee them the performance of industrial plants, which in some cases are even rented rather than bought outright (Vössing et al. 2019).
In mechanical engineering, this business model is already known as “machine-as-a-service.” Instead of selling industrial plants, this novel business model requires German SMEs to use their internal “service knowledge” (construction knowledge about the machine, upcoming operations and maintenance tasks, optimal machine configurations, and continuous wear and tear) to guarantee their customers the contractually assured availability or energy-efficient and material-efficient operation.
The “service knowledge” on industrial plants required for the provision of these high-quality services already exceeds the knowledge of even experienced service technicians and, in some cases, even of companies, due to the increasing complexity and advancing digitalization of machines and plants (e.g. due to the significantly higher proportion of mechatronics). Maintenance concepts within the framework of operator models not only require the implementation of classic, standardized maintenance intervals and task lists, but also require the combination of the most diverse sources of knowledge (i.e. customer production plans, sensor measurements on operation and wear, machine history, design) in order to adapt the deployment planning to the individual needs of individual machines. In particular, (future) problems of industrial plants can be identified not only by AI-based analysis of individual machines (“Predictive Maintenance”), but also by also AI-based, cross-customer analyses of machine data (“Peer Group Comparisons”).
However, performing such analyses requires competence in the operation of AI and big data analyses and exceeds the capabilities of service technicians. As the STEP research project showed, it is partly for these reasons that the “informal” exchange of knowledge among employees through chat applications such as Whatsapp and Facebook Messenger has greatly increased in practice. Due to the dearth of possibilities to bring this knowledge back into the company as well as the increasing lack of highly qualified employees, German SMEs are facing enormous challenges in the next few years to secure their leading edge in the provision of services.
In order to support German SMEs in compiling and providing the service knowledge necessary for operator models, a service platform that spans multiple plants, departments, and companies is required. This platform should, in particular, centralize the exchange of knowledge among technicians and also analyze employee communication, service documents, and sensor data in a legally compliant manner, with the aim of making the resulting “service knowledge” automatically available to technicians as “service intelligence.” Service Intelligence can support the sales and development departments in addition to the use of field staff.
The Sales department needs the knowledge to create machine-as-a-service offers in order to arrive at a viable cost calculation for the specific application (operational environment, capacity utilization, etc.). The Development department in turn needs the knowledge to design the next generation of intelligent industrial plants. Methods of artificial intelligence (AI) have already proven in practice and in research to be capable of enable service intelligence (Kühl et al. 2019). However, the existing methods are not yet sufficient to
- a) to support the service process throughout, and
- b) to be also available as a central service platform to small and medium-sized enterprises in a financially viable manner .
- c) Furthermore, existing AI approaches do not allow business users to interact with the data. Today, this requires specially trained analysts (data scientists), who provide the intelligence from the existing data.
Service-Meister will build this service platform and thus create the prerequisite for a service ecosystem, as it enables SMEs in particular to involve external partners in the provision of services for their customers. For example, Service-Meister will enable external staff to correct plate change errors on presses from a third-party manufacturer because they are intelligently supported by Service-Meister. Expert knowledge digitized by AI and Smart Services can be shared and made available worldwide, enabling other partners to independently offer high-quality services in the ecosystem. These AI-based systems on the Service-Meister platform also enable external services to be provided on the basis of the service platform. This is the only way to create a so-called service ecosystem for all participants. This new form of cooperation sustainably strengthens both SMEs and Germany as an industrial location.
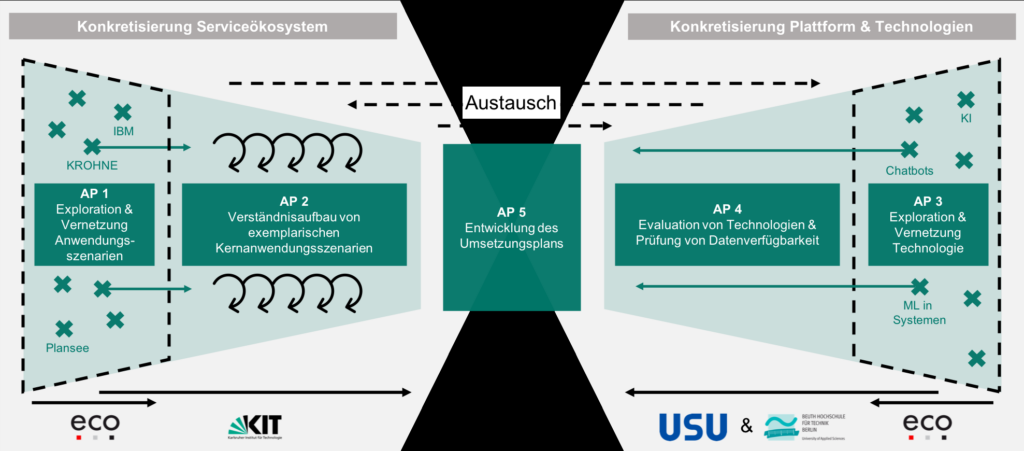
The Service-Meister project develops and validates such an AI-based service platform for German SMEs. By means of artificial intelligence and the Smart Services provided in Service-Meister, the complexity of service provision within the framework of operator models (e.g. machine-as-a-service) will be manageable. Despite limited staff resources, this sector can operate market-leading service offerings cost-efficiently and competitively by supporting the Smart Services on the Service-Meister platform, such as a digital advisor in the form of a ServiceBot. Service-Meister focuses on the following areas: (1) creation of a standard for easy information integration along the service process chain (Service XML) and for easy integration of partners into the service ecosystem (plug-and-play platform) (“Service XML is the OPC-UA for software!”), (2) the development of a cross-company and cross-machine platform that connects technicians with machines as well as technicians with each other, and (3) the development of AI-based services (e.g. ServiceBots) that allow technicians to interact with the available knowledge base and automate routine tasks (e.g., automatically understand and summarize thousands of service reports), creating digital guides for service personnel (Talk-To-Reports).
.